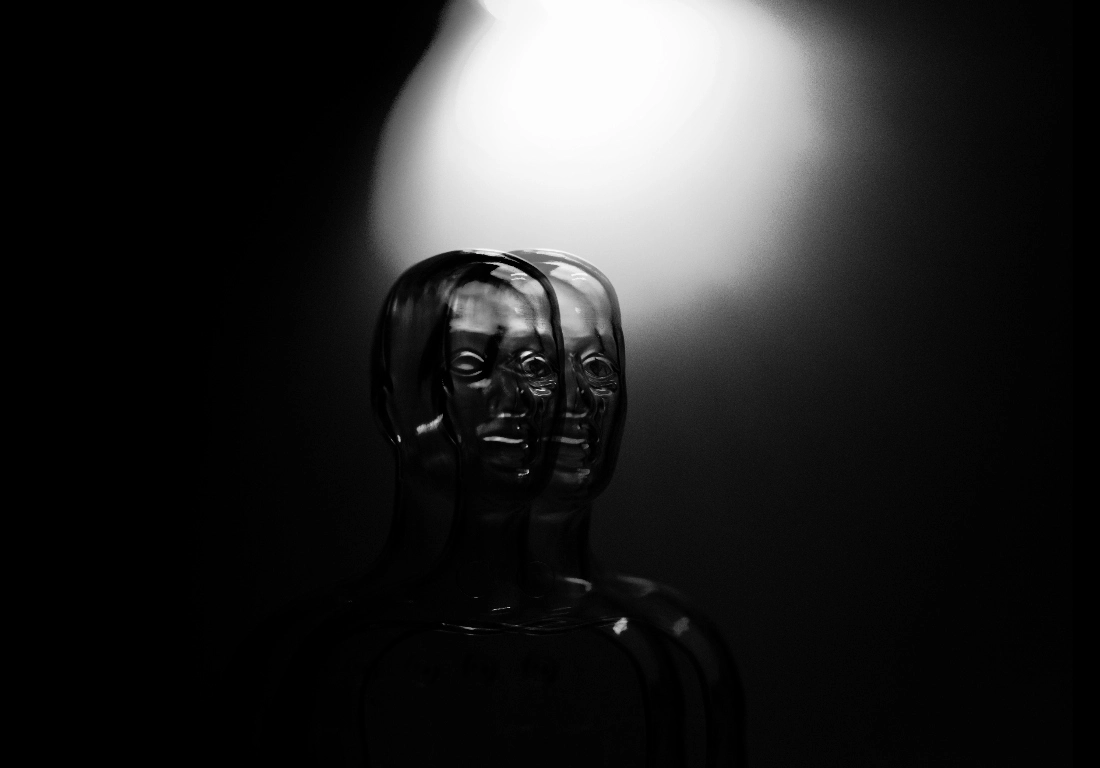
image source: www.pexels.com
Ever wondered how smart machines learn things? It’s like comparing the old school with the cool new kid on the block.
Traditional AI follows strict rules, while generative AI is all about machines learning and creating stuff on their own. This generative AI vs traditional AI article is like a friendly chat about these two AI pals, exploring what makes them tick and how they’re different.
So, imagine you’re on a tech adventure – which AI buddy would you want by your side?
Let’s dive in and find out how these smart technologies are shaping the future, and explore the difference between generative AI vs traditional AI!
What Is Generative AI?
Generative AI is like having a digital artist on your computer. It’s a type of artificial intelligence that’s designed to create new content, like images, music, or text, based on patterns it learns from existing data.
Think of it this way: Imagine you have a friend who’s a brilliant artist. You show them a bunch of paintings of flowers, and after studying them, they can create their unique flower paintings that look like they were made by a professional artist. Generative AI works kind of like that friend, but it’s a computer program.
One popular example of generative AI is Adobe Photoshop, especially with its Content-Aware Fill feature.
When you use Content-Aware Fill, you can remove objects from a photo, and Photoshop automatically fills in the background based on the surrounding pixels. It’s like magic! Photoshop uses complex algorithms to understand the content of the image and generate new pixels that seamlessly blend with the existing ones.
So, in a way, it’s creating new content based on what it’s learned from the original image data.
What Is Traditional AI?
Traditional AI refers to the older methods of artificial intelligence that typically follow a rule-based approach. Instead of learning from data like generative AI does, traditional AI relies on predefined rules and logic programmed by humans.
When it comes to generative AI vs traditional AI, the former is the one leading the future of artificial intelligence and machine learning technologies.
Imagine you have a puzzle with specific rules on how each piece can move. Traditional AI is like solving that puzzle by following a set of strict instructions step by step. It doesn’t learn or adapt; it just executes the commands it’s given.
A classic example of traditional AI is a chess-playing computer program like Deep Blue. Deep Blue was programmed with rules that govern how chess pieces move and strategies for winning the game. When you play against Deep Blue, it evaluates possible moves based on those rules and selects the best one according to its programmed algorithms.
In generative AI vs traditional AI, the latter doesn’t create new content or adapt to new situations.
It’s good at tasks where the rules are well-defined and the problem space is limited, like playing chess or solving mathematical equations. However, it struggles with tasks that require creativity, adaptation, or dealing with uncertain or messy data.
Key Characteristics of Generative AI vs Traditional AI
In the realm of artificial intelligence (AI), two prominent approaches stand out: generative AI and traditional AI. While both aim to solve problems and perform tasks, they do so through fundamentally different methods.
Generative AI relies on learning from data to create new content, while traditional AI follows predefined rules and logic. Understanding the key characteristics of these two approaches is crucial for grasping their applications and potential.
Characteristics of Generative AI:
Generative AI operates by learning from vast amounts of data and generating new content based on those patterns. It leverages techniques such as neural networks and deep learning to understand and replicate complex patterns within the data. This approach enables generative AI systems to create realistic outputs in various domains, including images, music, and text. One of the distinguishing features of generative AI is its ability to produce novel content that it has not explicitly encountered before. This creativity and adaptability make it suitable for tasks requiring innovation and imagination.
Characteristics of Traditional AI:
In contrast, traditional AI relies on predefined rules and logic programmed by humans. It operates based on a set of instructions designed to solve specific problems or perform particular tasks. Traditional AI excels in scenarios where the problem space is well-defined and the rules are clear.
Examples include chess-playing programs and expert systems for diagnosing medical conditions. However, traditional AI struggles with tasks that involve ambiguity or require adapting to new situations.
Characteristic | Generative AI | Traditional AI |
---|---|---|
Learning Approach | Learns from data to create new content | Follows predefined rules and logic |
Creativity | Generates novel content based on learned patterns | Executes predefined instructions |
Adaptability | Adapts to new situations and data | Limited adaptability, relies on fixed rules |
Application | Suitable for creative tasks and content generation | Effective for well-defined problems and tasks |
Example | Adobe Photoshop’s Content-Aware Fill | Deep Blue (chess-playing computer program) |
In summary, generative AI and traditional AI represent two distinct approaches to artificial intelligence, each with its own set of characteristics and applications.
Generative AI harnesses the power of data to create new content, showcasing creativity and adaptability. On the other hand, traditional AI operates within predefined rules, excelling in tasks with clear parameters.
Understanding the differences between these approaches is essential for leveraging their capabilities effectively in various domains.
Differentiating Between Orthodox & Modern Artificial Intelligence
1. Approach to Problem-solving:
Traditional AI: Traditional AI relies on rule-based methods, where explicit instructions and predefined rules are programmed to enable the system to carry out specific tasks. These rules are formulated by experts in the field based on their understanding of the problem. Traditional AI systems adhere to these rules to make decisions and produce results.
Generative AI: Generative AI adopts a data-driven approach. It learns from extensive datasets using techniques such as deep neural networks to identify patterns and structures. Instead of depending on explicit rules, Generative AI models glean insights from the data and generate fresh content by capturing inherent patterns and relationships within the dataset.
2. Learning Strategies:
Traditional AI: Traditional AI often employs supervised learning, where the AI model is trained on labeled data, providing inputs and their corresponding outputs. Through this process, the model learns to associate inputs with specific outcomes based on the labeled examples, relying on human annotations for accurate predictions.
Generative AI: Generative AI can utilize both supervised and unsupervised learning, excelling particularly in unsupervised scenarios. In unsupervised learning, the model is exposed to unlabeled data, enabling it to discern underlying patterns and structures without direct human guidance. This capacity to generate new data and content empowers Generative AI in unsupervised settings.
3. Model Approaches:
Traditional AI: Traditional AI predominantly employs discriminative models, which aim to distinguish between different categories or classes of data. For instance, in image classification, a discriminative model is trained to categorize images into specific groups (e.g., cats or dogs) based on their features.
Generative AI: Generative AI utilizes generative models, which grasp the underlying probability distribution of the data and can produce new samples resembling the original dataset. Notably, Generative Adversarial Networks (GANs) are a notable example of a generative model capable of generating realistic images resembling real-world examples.
4. Creativity and Adaptability:
Traditional AI: Traditional AI is tailored for specific tasks and lacks inherent creativity and adaptability beyond its predetermined programming. It operates within predefined rules and cannot generate fresh content or adapt to novel situations without direct human intervention.
Generative AI: In contrast, Generative AI showcases creativity and adaptability due to its capacity to generate innovative content. It can produce new images, texts, music, and more, offering unique and imaginative outputs. Moreover, Generative AI can adjust to diverse data distributions and generate content aligned with emerging patterns or variations in the input data.
Final Words: The Future of AI
While traditional AI and generative AI serve distinct purposes, they complement each other rather than operating in isolation. By integrating generative AI with traditional AI, we can enhance the efficacy of AI-driven solutions.
For example, traditional AI might scrutinize user behavior data, while generative AI could leverage this analysis to craft personalized content.
As we delve deeper into the vast possibilities of AI, it becomes imperative to grasp these nuances. Both generative AI and traditional AI wield substantial influence in shaping our future, each offering its array of opportunities. Embracing these cutting-edge technologies will prove essential for businesses and individuals striving to maintain a competitive edge in our swiftly evolving digital terrain.
Our expedition into AI innovation has just commenced. Acknowledging the distinctive strengths of these AI variants empowers us to fully exploit their potential as we embark on this thrilling voyage.
People Also Read:
ChatGPT Alternatives: 6 Best AI Marketing Tools In 2024
How ethical is AI and things you need to know.
Can AI Replace Marketing Jobs Soon?
Top 10 AI Tools For Business Productivity.